Meet the 10 up-and-coming researchers selected to participate in WiGRAPH’s inaugural Rising Stars program, a two-year program of mentorship and workshops co-located with SIGGRAPH 2022 and 2023 to explore potential career trajectories as they enter the job market.
Click on any of our Rising Stars to learn more about them!
Meet Lizeth Fuentes
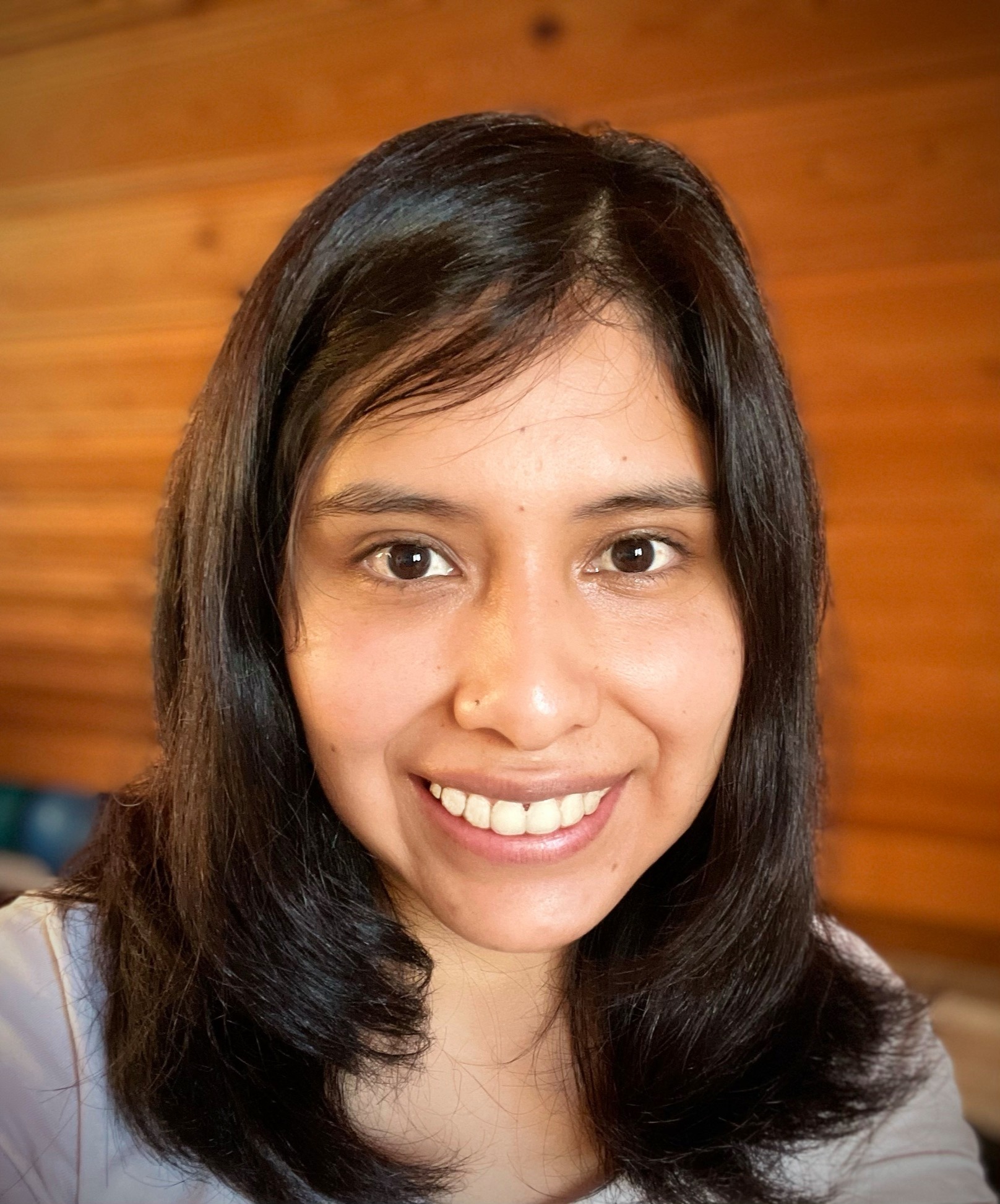
Research Vision: Humans take for granted the process of perceiving a scene and understanding its high-level structures. Nevertheless, this is a challenging problem for a computer. A standard way to discretize real environments is through accurate measurements of the 3D space, also known as point cloud data. Working with this data is challenging due to the lack of annotations (e.g., floor, ceiling, walls), high redundancy, uneven sampling density, noise, outliers, and especially their unstructured nature. The automatic reconstruction of indoor environments is particularly challenging because the data also present higher occlusion levels, and each indoor scan can reach hundreds of millions of points. Also, a scene has several instances of unknown categories, so imposing strong assumptions (priors) is the de facto standard to reduce the complexity of this problem.
The general goal of my research project goes beyond surface reconstruction; it intends to reconstruct a simplified CAD model, which contains semantic information such as structural and dynamic elements and the relationships between them. To this end, we are working on learning techniques to subdivide the scene into meaningful parts instead of simple planar primitives. The overall goal is to combine semantically consistent parts to reconstruct the 3D scene.
Bio: Lizeth was born in Arequipa, Peru. She is currently a PhD student in Computer Science at the University of Zurich and an early-stage researcher in the EVOCATION innovative training network. She has been awarded the Grace Hopper Student Scholarship to attend the world's largest gathering of women technologists GHC 2020. Prior, she received her MSc in Computer Science from the Federal Fluminense University, Niteroi - Brazil (2017). She earned her BSc in Computer Science from the National University of Saint Augustine, Arequipa - Peru (2014). Her research interests are geometry processing, computer graphics, computer vision, and machine learning.
Meet Maria Larsson
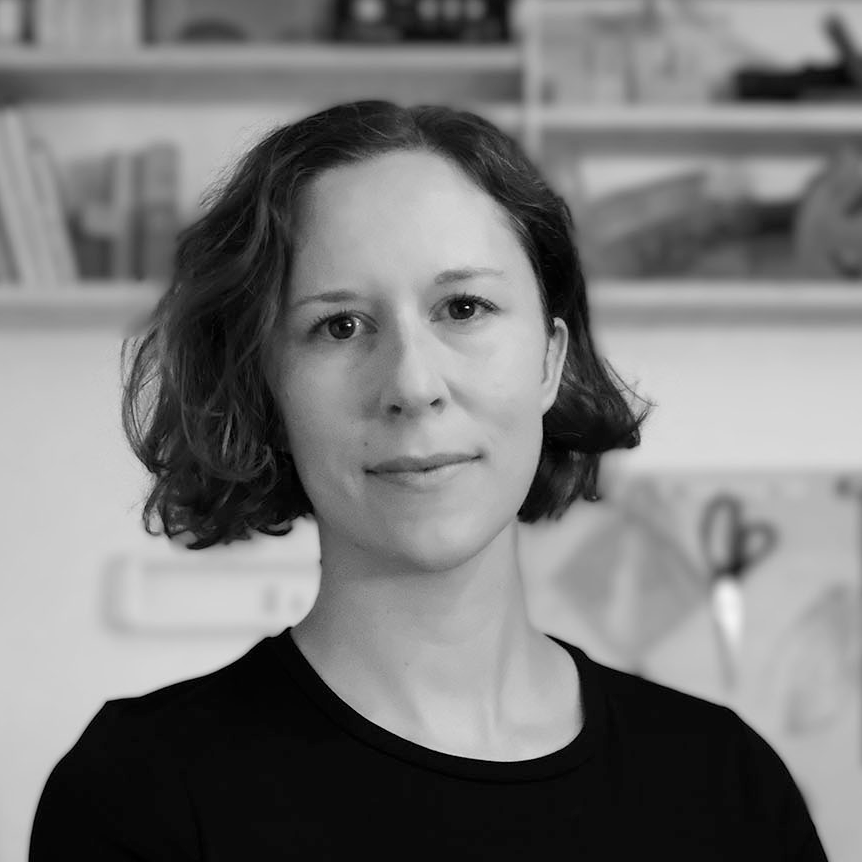
Research Vision: My research aims to enhance human creativity via technology. Starting out as an architectural designer specializing in parametric 3D modeling, I became increasingly interested in developing more scalable design and visualization tools, which motivated me to turn to computer graphics and user interface design. Drawing inspiration from traditional practices and materials of the physical world, I aim to develop digital tools and systems that facilitate the creation of artifacts and structures, while paying particular attention to sustainable and efficient use of material resources. Specifically, I am interested in wood material, carpentry (a reason to live in Japan!), computer numerical control (CNC) manufacturing, and augmented reality interfaces for construction.
Bio: Maria Larsson is a PhD Candidate at the User Interface Lab, The University of Tokyo, advised by Professor Takeo Igarashi. She is also a Research Assistant at the Research Institute for an Inclusive Society through Engineering (RIISE) and a recipient of the ACT-X fellowship from the Japanese Science and Technology Agency (JST). She is the developer of Tsugite, an interactive tool for designing and fabricating wooden joinery, which received significant media attention, including Le Monde and Desginboom. She has a background as an architect designer with several years of professional experience, working for Pool Architekten in Switzerland and the Digital Design Lab of Nikken Sekkei in Japan. She received an M.Eng. in Architecture from The University of Tokyo, and a B.A. in History of Art and Architecture from Harvard University. She is a former NCAA Fencing Team Champion and her hobbies include crafts and camping.
Meet Mackenzie Leake
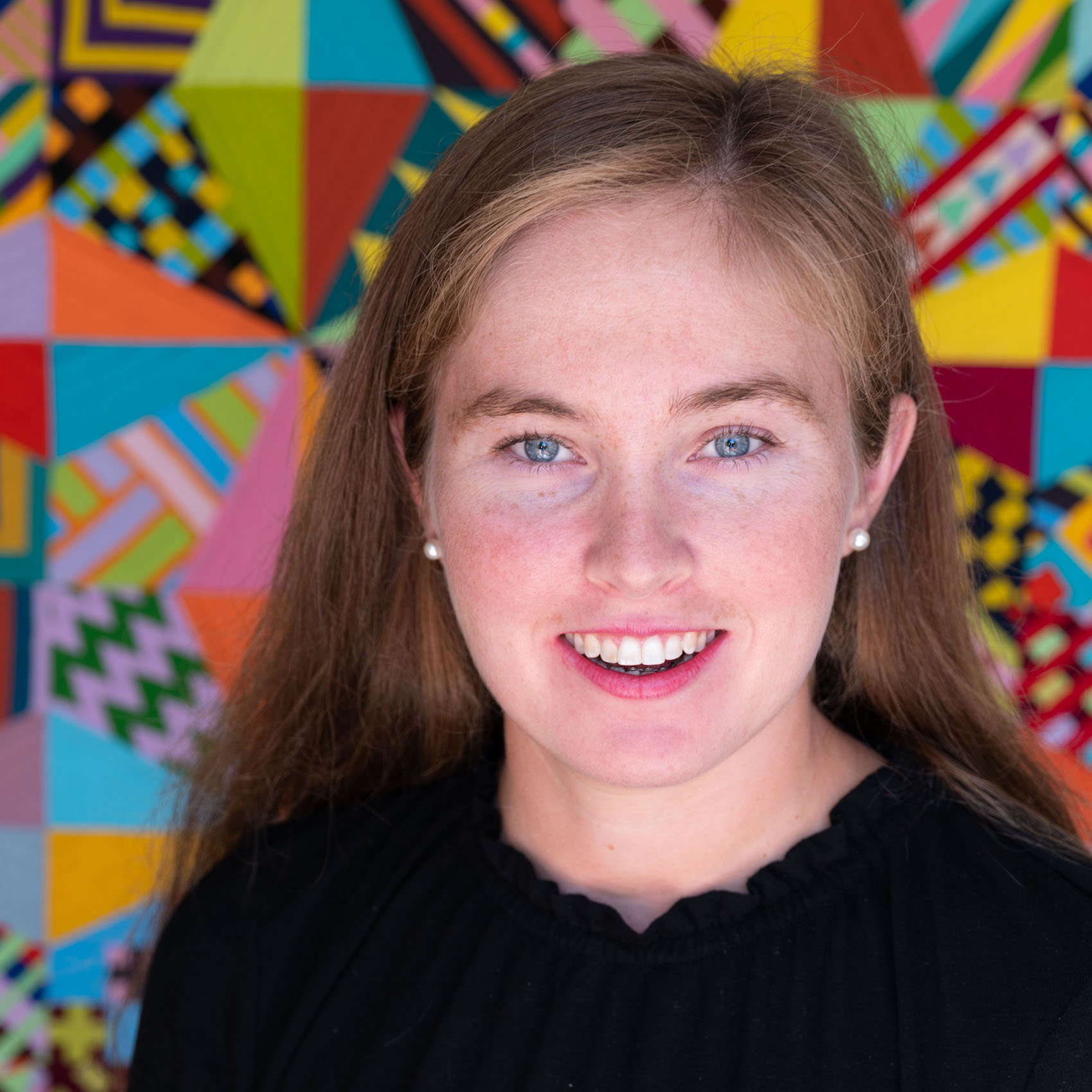
Research Vision: Creative tasks often require practitioners to navigate a large space of options for each design decision. While some of this navigation may be essential exploration, some tasks can distract from the more important creative decisions. In my research I develop tools that enable users to focus on the creative parts of their process while offloading some of the tedious parts to computational tools. I apply a combination of approaches from HCI and graphics to develop algorithms and systems to record, represent, and aid creative processes in domains, such as quiltmaking and video editing. Well-designed tools have the power to help users realize their creative visions, and in order to support this, we must understand existing craft processes and make it easy for users to express their design intentions. My broader goal is to further our understanding of how computation can support and extend our creative capabilities.
Bio: Mackenzie Leake is a METEOR postdoctoral fellow at MIT CSAIL. She received her PhD and MS in computer science from Stanford University and a BA in computational science and studio art from Scripps College. Her research focuses on designing computational tools for various creative domains, including textiles and video. Her research has been supported by Adobe Research, Brown Institute for Media Innovation, and Stanford Enhancing Diversity in Graduate Education (EDGE) fellowships. Her research has been published at several computer graphics and HCI venues, including SIGGRAPH and CHI, and her work has been covered in various news outlets, including Wired, CinemaEditor, and Engadget. She is active in the modern quilting community, and her quilts have been exhibited at the Houston International Quilt Festival, the AQS Paducah Quilt Show, and QuiltCon.
Meet Chenxi Liu
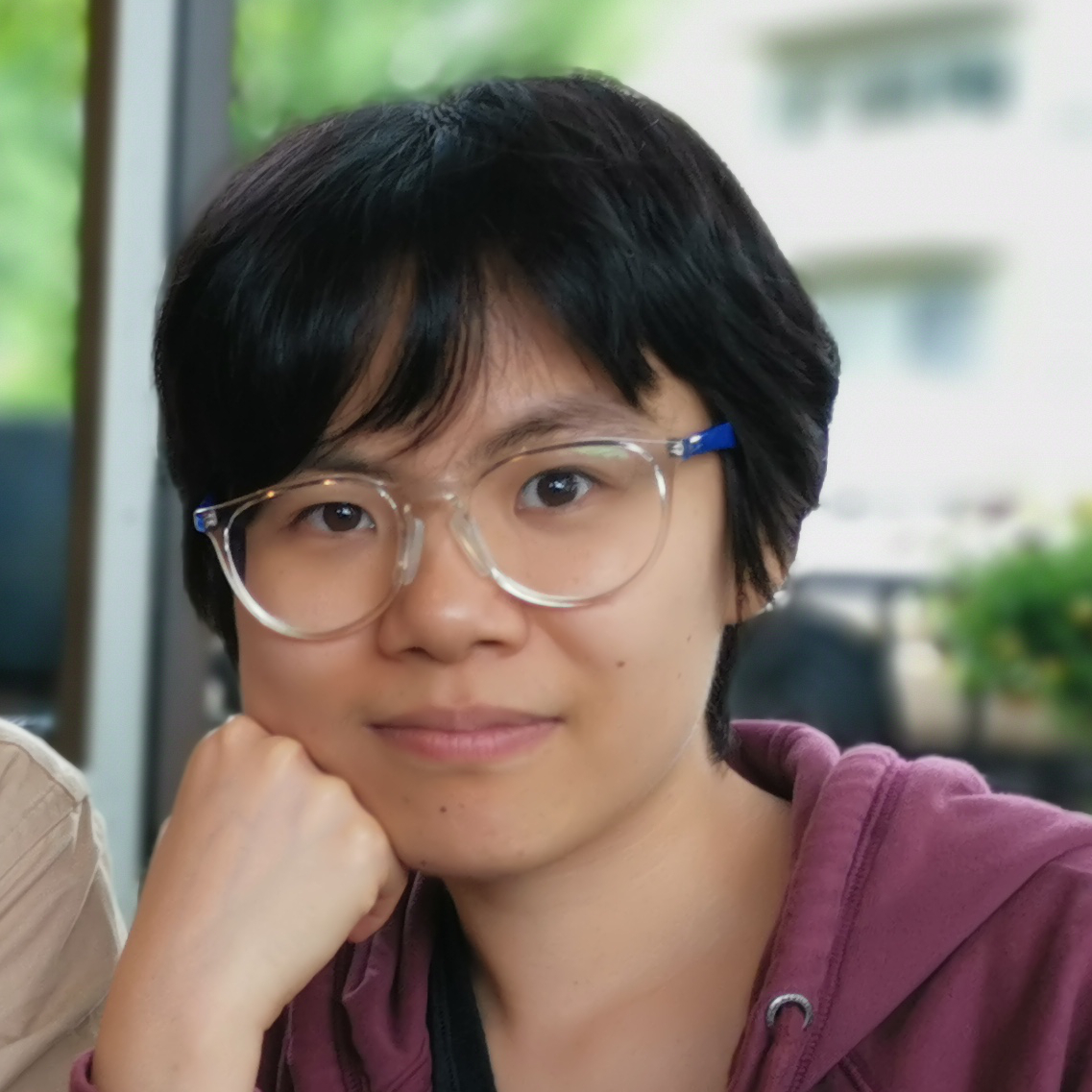
Research Vision: My passion lies in computationally understanding and creating line drawings. Although with recent progress in Vision and Deep Learning, automatic methods are making new achievements on a daily basis in visual recognition and generation, these current developments are mainly pixel-based. Reliable intelligent creation tools for tasks where structures of drawings are essential, such as vector drawing, sketch-based modeling, and coloring 2D art, still do not exist. My PhD research consists of attempts to bridge rough freehand line drawings with precise digital representations and also to robustly and accurately generate line drawings based on 3D models. For the future, my goal is to better understand as well as assist sketching and drawing in general.
Bio: Chenxi Liu is a PhD candidate at University of British Columbia, advised by Prof. Alla Sheffer. Her research focuses on line drawing processing and generation of stylized images from digital models. Chenxi received her M.S. at Carnegie Mellon University and her B.Eng. at Beihang University in China. She has previously interned at Adobe Research and Disney Research.
Meet Karima Ma
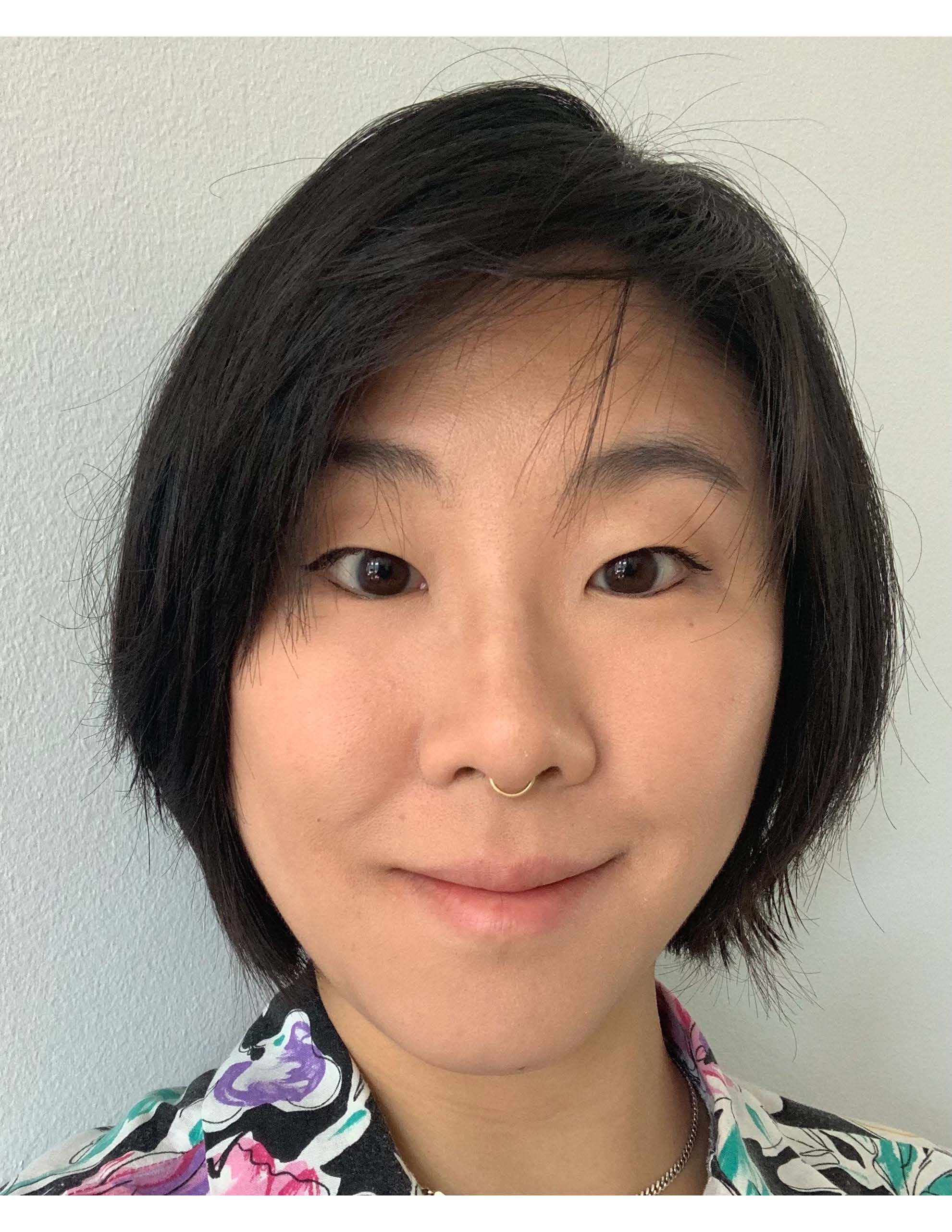
Research Vision: I am a PhD student at MIT advised by Jonathan Ragan-Kelley. I focus on domain specific programming and program optimization for image processing tasks. I work at the intersection of machine learning, graphics, compilers, and systems research. My research uses machine learning to search over programs to automatically optimize the runtime performance and output quality of image processing applications. I am interested in designing systems that fuse domain specific knowledge of image processing and high performance computing with machine learning. My goal is to build systems that make generating efficient and high quality signal processing applications for various hardware targets easy and accessible instead of relying on rare interdisciplinary experts to engineer bespoke programs.
A key challenge in my work is defining the search space for these problems so that they are tractable, but broad enough to yield good programs and generalize to a range of applications. These search spaces are challenging to optimize over because they contain continuous parameters and discrete structures, but they are increasingly relevant in graphics research as we move more applications towards differentiable programming. I am interested in how we can inform program synthesis and machine learning systems with domain specific knowledge to produce better programs than human experts or expensive black box models alone can.
Bio: Karima is a PhD student at MIT in the Computer Science and Artificial Intelligence Lab (CSAIL). Prior to embarking on her PhD, she obtained a Masters in Computer Science at Carnegie Mellon University and majored in Economics and minored in Computer Science and Chinese Language and Literature at Dartmouth College. She did not know how to code until her junior year in college, which goes to show that it's never too late! During her PhD she has enjoyed collaborating with researchers in industry at Adobe Research and Facebook AI Research. In her free time she tries to stay away from her computer with various activities which include but are not limited to rock climbing, drawing, writing, and enjoying live music.
Meet Siqi Wang
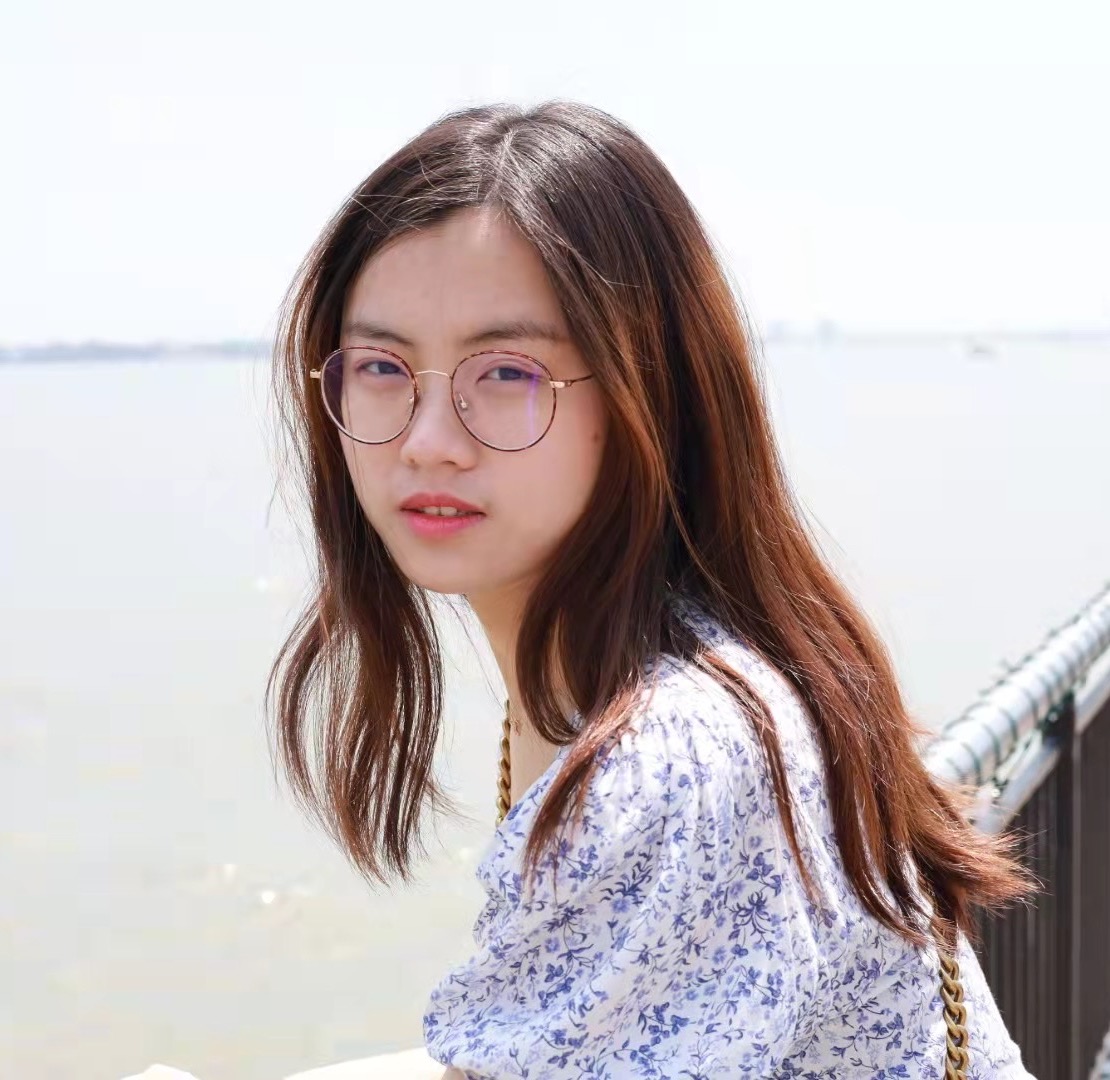
Research Vision: My research interest in computer graphics lies in physical simulation, geometric computing and the interdisciplinary studies of the two. My research addresses physical simulation problems using finite-difference method (FDM), finite element method (FEM) or material point method (MPM) and their broad applications in high performance computing and fabrications. The first topic of my research is to bridge the gap between virtual and real-world visual effects, involving high-fidelity Eulerian anisotropic fluid simulations and appearance-preserving tactile simulations with the help of shape optimization. The second topic emphasizes the importance of simulation quality and efficiency with geometry processing approaches, such as adaptive refinements on both triangle and tetrahedral meshes. I am also exploring the use of refinements in warping scalable vector graphics and considering developing data-driven simulation algorithms to model the physical interactions of the virtual world in the future.
Bio: Siqi Wang is a third-year PhD student in Computer Science at New York University, advised by Prof. Denis Zorin and Daniele Panozzo. Prior to that, she completed her B.S. degree in Electrical Engineering from Shanghai Jiao Tong University in 2019. Currently, she is working as a research scientist intern at Adobe Research, under the mentorship of Alec Jacobson. She received DeepMind Scholarship in 2021 and MacCracken Fellowship from NYU in 2019. She attended SIGGRAPH virtually in both 2021 and 2020. Her research in physical simulations and geometry computing aims to improve the accuracy and efficiency of the physical world modeling, with wide applications in high performance computing and fabrications. After work, she is also an ameteur pianist, a ballet beginner and a video games and comics lover.
Meet Stephanie Wang
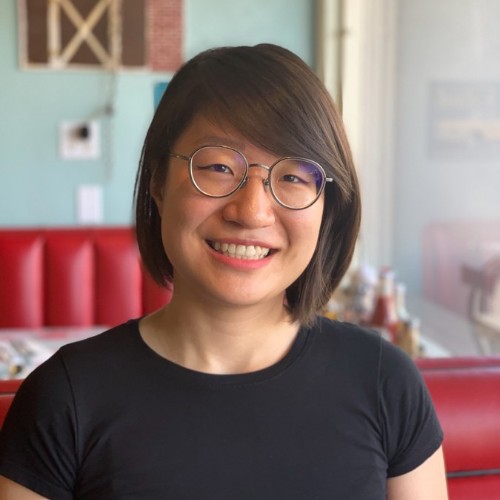
Research Vision: My research interests lie in partial differential equations and optimization with applications in geometry processing and physical simulations. I find myself gravitating towards research topics that have a real-world impact while also allowing me to apply my pure math training. In geometric processing, optimization with geometries is a combined effort of geometric representations, differential equations, and optimization methods. In simulations for a virtual world, physics models and the differential equations of their time-evolution are the prominent research topics. Mathematical theories can serve to provide novel discretization schemes that are faster and conserve important physical quantities better.
Bio: Stephanie Wang is a postdoc at UC San Diego working with Professor Albert Chern. She received her PhD (2020) in Mathematics from UCLA with a dissertation on physics simulation of various materials using the Material Point Method, advised by Professor Joseph Teran. She received her Bachelor's degree (2013), also in Mathematics, from National Taiwan University. She was the leader of Woman in Math at UCLA and the creator of the Woman in Math Mentorship Program. In her free time, she is an avid hiker and loves to cook.
Meet Jane Wu
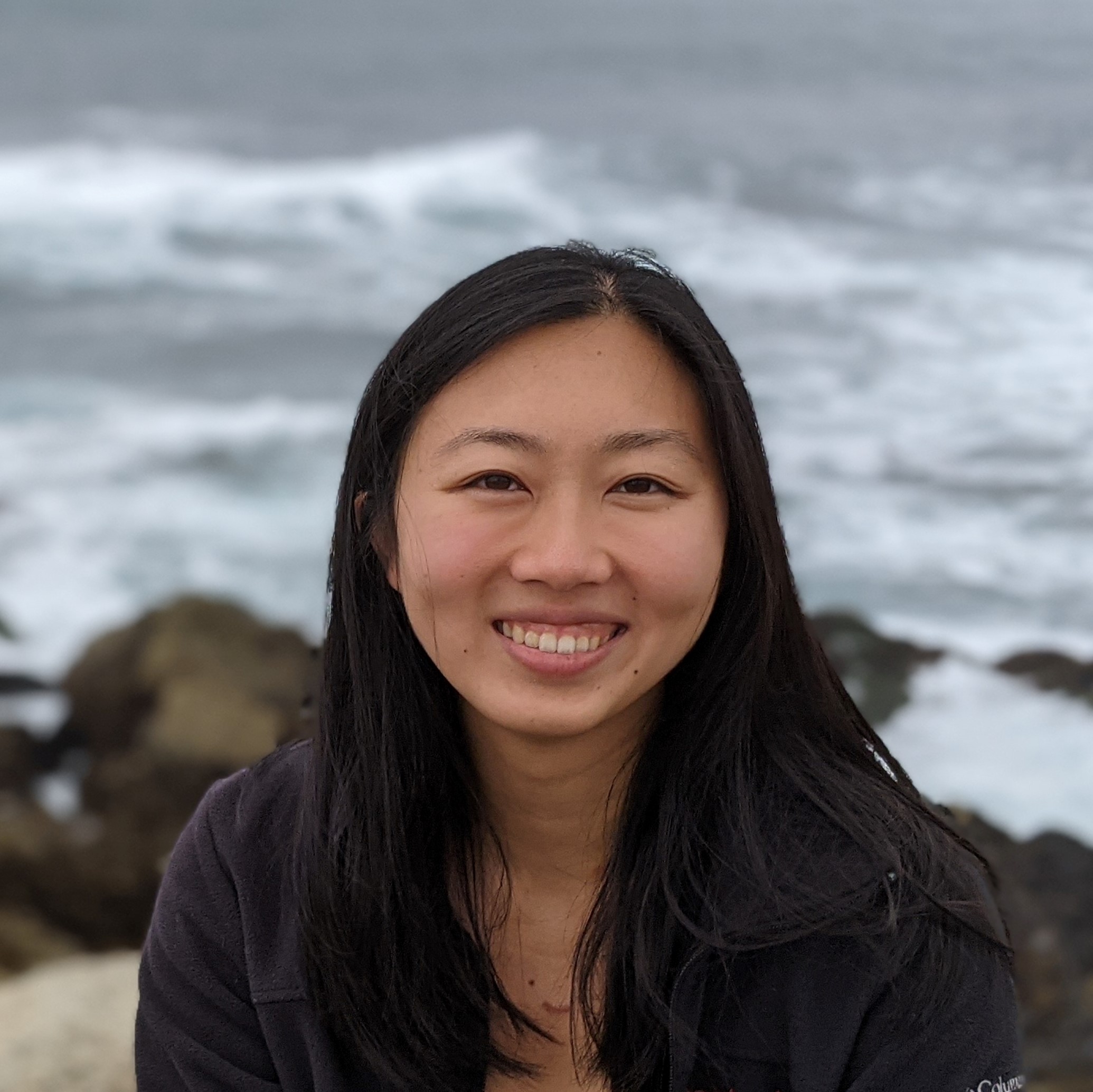
Research Vision: My research interests lie at the intersection of computer vision, graphics, and physical simulation. The overlap between these three areas is rapidly growing in computer science, and I believe there is a synergy between incorporating graphics principles in computer vision algorithms and deep learning-based simulation and rendering to accelerate real-time graphics systems. My recent work in cloth shape estimation demonstrates the efficacy of learning high-frequency information embedded in low-frequency geometric structures. The first parameterization we developed is texture sliding, a method for recovering cloth geometry by learning deformation using two-dimensional camera projections onto texture space. The second parameterization is a tetrahedral mesh framework constructed by skinning the volume of air around the body. Cloth can then be embedded in the tetrahedral mesh, and for each pose the cloth shape is predicted by training a deep neural network to learn offsets from the rest pose cloth embedding. Building on these data representations, I am currently developing a real-time cloth capture system towards the goal of low-cost solutions for human digitization. While advances in graphics/simulation have proven useful for many state-of-the-art computer vision algorithms, I believe that problems in graphics, such as accelerating large-scale simulations, can equally benefit from advances in machine learning.
Bio: Jane Wu is a PhD student in Computer Science at Stanford University, advised by Professor Ron Fedkiw. Her research interests lie at the intersection of graphics and computer vision, including clothed human reconstruction, neural rendering, and physics-based machine learning. For her PhD thesis, she has been investigating methods for predicting high-frequency geometric details via low-frequency embeddings for the application of cloth capture. Jane is also a part-time R&D software engineer at NVIDIA and she was previously a research intern at Google and NVIDIA. She is a recipient of the Gerald J. Lieberman Fellowship. Jane was a co-chair of Stanford Graduate Women in CS (WiCS) from 2019-2021 and she co-organized the Stanford-Berkeley Research Meetup for Women in CS/EE in 2022. Of her hobbies, she especially enjoys playing the violin and has been a member of the Stanford SSO and SP orchestras.
Meet Mengqi (Mandy) Xia
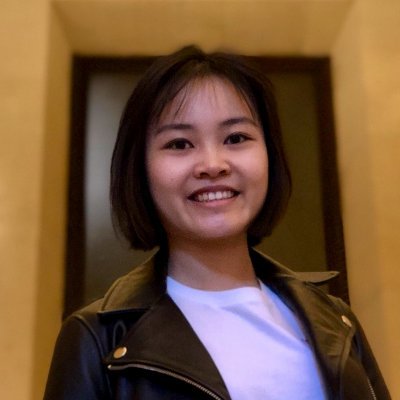
Research Vision: My research interest lies in physically based rendering and I’m excited about applying optics and Electromagnetics theories to realistically reproduce the appearance of complex materials. During my PhD, I have worked on different types of materials including fibers, human skin, surfaces, and discrete media. In particular, I have worked on applying wave optics to model fiber scattering to more realistically render human hair, fur and textile fibers and applying wave optics to render discrete water droplets, in combination with physics-based simulation. These research experiences motivate me to identify the missing components in material modeling. In the future, I envision applying wave optics theory as a solid foundation for building a novel rendering system. My research will also involve making the aforementioned system differentiable and suitable for inverse problems and applying it to applications such as nanostructure fabrication and medical imaging. Another part of my research will be devoted to integrating rendering and simulation for developing next-generation material models. I believe this research can significantly improve the realism of digital reproductions of the physical world.
Bio: Mandy Xia is a PhD student in computer science at Cornell University where she is advised by Professor Steve Marschner. She works on physically based rendering and studies how light interacts with complex materials. She received her Bachelor's degree in Applied Mathematics from University of California, Los Angeles (UCLA) with Summa Cum Laude. She was a selected participant for Rising Stars in EECS 2020. She will join as a postdoc researcher in professor Wenzel Jakob’s research group at EPFL starting Fall 2022. In her spare time, Mandy loves rock climbing, hiking and camping.
Meet Emilie Yu
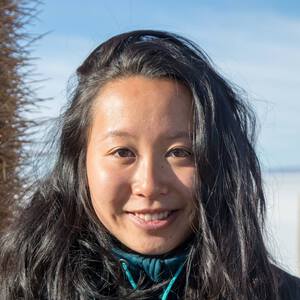
Research Vision: My research interests revolve around developing computational methods that are intertwined with user interactions, to make ill-posed problems tractable and provide control over the result. I am most interested in creativity tools, such as for 3D design, 3D painting or sketching. I try to approach problems from both a graphics and human computer interaction perspective, to design tools for artists that enable new workflows and practices. During the start of my PhD, I focused on how VR sketching can be used as a 3D surface design tool, now I am interested in scenarios where digital 3D sketches can be mixed with real captured footage, and in the future I would like to explore the emerging medium of 3D painting with VR tools, from the perspective of practicing artists.
Bio: Emilie is a second-year PhD student on the GraphDeco team at Inria and the Université Nice Côte d'Azur, advised by Adrien Bousseau. Previously, she obtained a joint Master’s in Computer Science from CentraleSupélec and in Computer Graphics from the Technical University of Denmark. She is currently interning at Adobe under the mentorship of Rubaiat Habib Kazi and Wilmot Li.